1 Role of AI in the Pharma & Biotech Industry
Drug Discovery & Design
Clinical‑Trial Optimization
Manufacturing & Process Control
Regulatory Compliance & Pharmacovigilance
6 Future Trends of AI in the Pharmaceutical & Biotechnology Sector
7 Challenges of Implementing AI in Pharma & Biotech
8 Why Should Pharma & Biotech Companies Invest in AI Technologies
9 The Pros and Cons of AI in the Pharma & Biotech Industry
Examples of AI Implementations in Pharma & Biotech Industry
11 Accelerate Your Pharma & Biotech Industry with AI‑Powered EQMS Software
12 What an AI‑EQMS brings to the table:
13 Conclusion
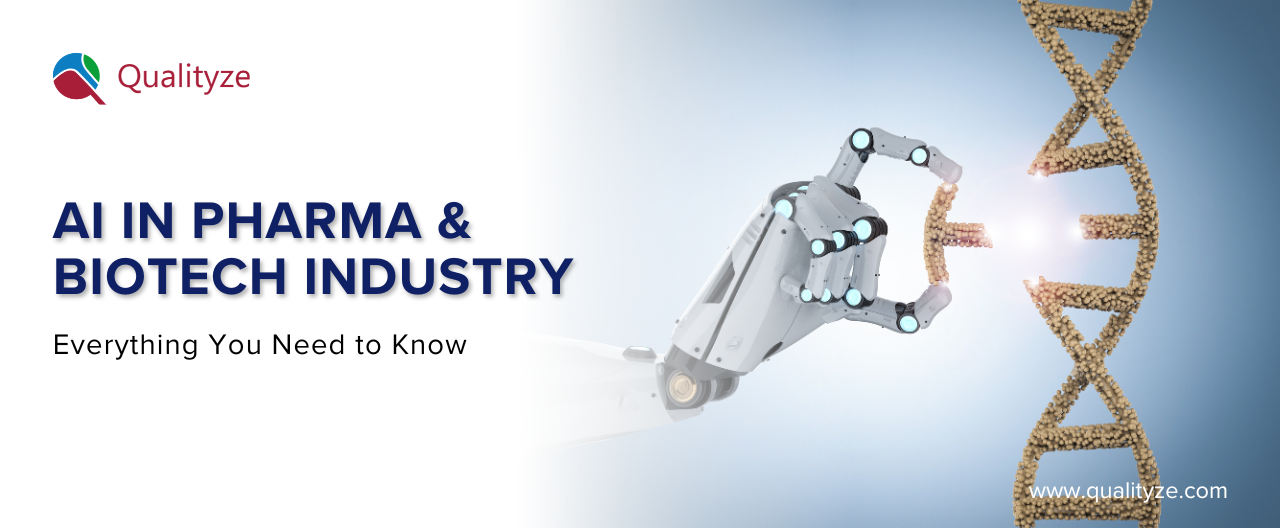
AI can turn months of guesswork into days of clear answers—helping pharma and biotech teams bring better medicines to patients sooner, with less risk and less waste.
Every pharmaceutical company, or precisely their R&D teams, are constantly brainstorming better ways to bring better drugs to market faster—without tripping over compliance. AI is finally giving them the keyboard shortcuts they’ve craved. From AI in pharma & biotech search analytics to real‑time deviation alerts on the plant floor, there is a revolution in a 150‑year‑old industry re‑compile itself in real time.
Two quick reality checks:
- Economic gravity is on your side. McKinsey’s latest life‑sciences deep dive says generative and analytical AI combined could unlock up to $110 billion in productivity across discovery, clinical, and commercial domains .
- Competitive pressure is rising fast. Gartner expects that by 2025 over 50 % of manufacturers will embed AI in quality control, lifting defect‑detection rates 30 % .
AI isn’t hype anymore; it’s hard ROI. McKinsey pegs the upside at US $60–110 billion a year for life‑science companies, while the market for AI platforms in pharma is compounding at 42.7 % through 2030. Early adopters are already halving discovery timelines, recruiting trial patients 15× faster, and chopping unplanned downtime on the shop floor by up to 50 % . In the blog that follows, we will look at the places where the wins are, where the potholes hide, and why an AI‑powered EQMS may be the most practical on‑ramp to this new era of quality‑driven growth.
With that in mind, let’s walk the pipeline—from molecule sketch to market surveillance—and pinpoint where AI delivers the biggest bang today.
Role of AI in the Pharma & Biotech Industry
AI is quietly reshaping every corner of the life‑science world. From the moment a chemist sketches a new molecule to the day regulators review safety updates, smart algorithms now lend a hand. Below is a plain‑spoken look at how this technology shows up in four big areas of work—without the jargon, but with solid evidence for each point.
Drug Discovery & Design
AI tools can scan huge digital libraries of chemicals and spot promising drug “hits” long before any lab work begins. Researchers at Atomwise have already demonstrated that approach on hundreds of different protein targets, proving the idea works across many disease areas. Once a short list of candidates is ready, structure‑prediction models such as AlphaFold help scientists see how each molecule might fit its protein target—almost like having X‑ray eyes for biology. By connecting these two steps, teams can drop weak leads early and move strong ones forward faster than ever.
Clinical‑Trial Optimization
Finding volunteers who match strict trial rules has always slowed new medicines. AI now combs electronic health records and other data to highlight people who truly qualify, so researchers spend less time screening. Science 37, for example, uses this approach to line up suitable participants and confirm their records before the first visit. Once a study begins, adaptive‑design software watches incoming results and can suggest small adjustments—such as changing dose levels or shifting the mix of patient groups—to keep the trial on track. These tools turn heavy, one‑size trials into flexible, data‑guided journeys.
Manufacturing & Process Control
On the production floor, computer‑vision cameras linked to neural networks now spot tiny cracks, fill mistakes, or label issues that the human eye might miss. Several large drug makers pairing this vision tech with cloud analytics have reported far fewer rejected units and steadier output. In another corner of the plant, predictive‑maintenance models listen to pumps, reactors, and filters, giving advance warning when something needs service—so equipment is fixed during scheduled breaks instead of causing a sudden shutdown. Together, these systems help teams make medicine more reliably and with less waste.
Regulatory Compliance & Pharmacovigilance
Regulators are watching AI closely and have begun publishing clear guidelines that spell out how companies must test, log, and update their models. Inside safety offices, specialized algorithms skim real‑world data—health‑care records, social media, and scientific papers—to catch early signs of side effects that might otherwise stay hidden. When a true signal emerges, automation scripts can pre‑fill large parts of required safety reports, freeing experts to focus on medical judgment rather than repetitive data entry.
In short, AI across drug discovery, trials, manufacturing, and compliance offers practical help right now. It finds ideas, lines up patients, guards the factory line, and keeps regulators informed—making every day work in pharma and biotech simpler and more dependable.
Future Trends of AI in the Pharmaceutical & Biotechnology Sector
- Explainable AI (XAI). Black‑box excuses won’t fly after the next 483 letter. Expect regulators to demand model cards and causal maps—think “audit trail meets Shapley values.
- Digital Twins. From virtual bioreactors that test 500 parameter tweaks overnight to patient‑specific disease models that simulate trial arms, twins will rewrite scale‑up and study design.
- Edge AI & 5G. Portable diagnostic scanners already analyze thermal breast images on‑device in rural India—proof that real‑time, low‑latency analytics beat spotty connectivity.
- Federated Learning. Privacy‑first AI collates insights without shipping PHI off‑prem, a must for GDPR‑risky cross‑border trials.
- AI‑driven Personalized Medicine. Adaptive protocols that tweak dosing per patient genotype will graduate from academic poster to standard of care within the decade.
Challenges of Implementing AI in Pharma & Biotech
- Data Quality & Interoperability. Siloed LIMS, MES, and legacy SAS datasets derail model training. FAIR data engineering—not shiny dashboards—is your real day‑one job.
- Regulatory Hurdles. The new FDA draft makes post‑market model drift monitoring mandatory; skipping it is liability roulette.
- Talent Gap. Only about 10 % of PhD‑level data scientists speak both Python and pharmacology according to Deloitte’s life‑sciences talent pulse .
- Ethics & Privacy. Federated learning and differential privacy are lifesavers, but you still need rigor around informed consent and algorithmic bias.
- Change Management. Front‑line scientists may eye the black box with suspicion. Quick wins plus open demo days help shift the culture.
Why Should Pharma & Biotech Companies Invest in AI Technologies
Artificial intelligence is quickly becoming the new operating system for life‑science work. Companies that weave it into everyday tasks discover they can move faster, work smarter, and stay in step with regulators. Here’s why that matters, explained in clear, simple language.
Faster Time‑to‑Market
AI tools can scan huge scientific data sets, suggest promising molecules, and help researchers make better choices earlier in the process. When the best ideas rise to the top sooner, medicines reach patients sooner.
Higher Trial Success Rates
Smart algorithms match trial designs to the right volunteers and spot potential problems before they derail a study. The result is fewer false starts and a smoother path from first‑in‑human to final approval.
Regulatory Peace of Mind
Modern compliance platforms powered by AI keep detailed, tamper‑proof records automatically . Inspectors see clear audit trails, and quality teams spend less time hunting for paperwork and more time improving processes.
Scalable Infrastructure
Because today’s AI systems live in the cloud, a company can start small, add new projects as confidence grows, and never worry about costly hardware replacements. It’s “pay as you need,” not “buy and hope.”
Competitive Advantage
Early adopters are already using AI insights to guide big decisions, cut down on guesswork, and out‑innovate slower competitors. Waiting on the sidelines is a risky bet in the fastest evolving market.
The Pros and Cons of AI in the Pharma & Biotech Industry
Pros | Cons |
Accelerated molecule discovery | High upfront investment & integration complexity |
Better predictive safety & efficacy models | “Black‑box” explainability worries |
Automated quality & compliance workflows | Data‑governance and privacy risks |
Personalized treatment regimens | Evolving, sometimes ambiguous, regulatory guidance |
Continuous process improvement via real‑time monitoring | Talent and change‑management hurdles |
Examples of AI Implementations in Pharma & Biotech Industry
- Atomwise leveraged deep learning to secure novel hits for 235 out of 318 validated targets—a leap over traditional HTS.
- Novartis × Microsoft employed computer vision to cut vial‑defect rejects by ~35 % and speed root‑cause analysis.
- Science 37’s decentralized OS clocks 15× faster enrollment and 3× more diverse cohorts, proving AI’s patient‑centric edge .
- GSK slashed site‑selection cycles to under 60 days via AI‑enabled protocol matching .
- Pfizer + IBM Watson mined immuno‑oncology biomarkers years ahead of manual approaches .
- Koerber Digital cites Gartner data showing AI‑driven QC lifts detection accuracy by 30 % while trimming false rejects up to 90 % .
These companies are actually operating‑budget line items delivering measurable gains.
Accelerate Your Pharma & Biotech Industry with AI‑Powered EQMS Software
Let’s talk brass tacks. You can chase siloed point solutions—or you can drop a single AI‑powered EQMS solution layer that stitches discovery data to shop‑floor batch records and post‑market surveillance.
What an AI‑EQMS brings to the table:
- Intelligent Document Control. Auto‑classification and e‑signatures keep ISO 13485 and 21 CFR Part 11 auditors smiling.
- Predictive Risk Dashboards. Machine‑learning models crunch trend data from deviations, CAPAs, and supplier audits, flagging red zones before they erupt.
- Adaptive Workflows. Think GitHub actions for quality: non‑conformances auto‑escalate if risk scores spike, while low‑risk items close on documented evidence.
- Unified Analytics. No more swivel‑chairing between LIMS, MES, and PLM; analyze the lot in one pane.
Bottom line? An AI Powered EQMS like Qualityze is the pragmatic gateway that lets quality teams ride the AI wave without reinventing every wheel in the organization.
Conclusion
AI is no longer the shiny object on a PowerPoint slide. It’s the gearbox transforming discovery sprints, clinical workflows, and quality baselines all at once. Companies capturing the upside are doing three things right now:
- Starting where the data is richest—often quality and manufacturing.
- Building explainability in from day one, not bolting it on for audits.
- Scaling with an AI‑ready EQMS, so every team speaks the same digital language.
Ready to see how it works for you? Book a 30‑minute live demo of our AI‑powered EQMS and walk through real dashboards, predictive alerts, and compliance reports tailored to pharma & biotech use cases.
Request Your Demo Now and turn AI from buzzword to business advantage.
Pass this story along to any colleague who still thinks AI in pharma is futuristic—they’ll thank you when their next audit sails through.
Share
The Start of Something Amazing.
Request Demo
Products
Industries